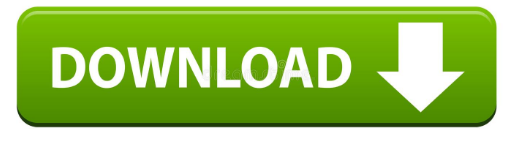

I would reassess how and why I chose to use these three, and possibly perform some exploratory data analysis beforehand using additional predictors. In this case, you don't have a large amount of predictors (only 3), but the fact that none boast predictive power to your response variable is concerning. (The former is an incredibly brief summary of a nuanced debate.) Some argue that dredging is the most empirical method, others find the 'whole kitchen sink' approach to be unacceptable as it doesn't account for ecological knowledge and is likely to result in overfitted models. There is significant controversy about how to best go about model selection see Analysing Ecological Data by Zuur (2011) for a life sci-related discussion. Since your "best" model is blank for all three, your summary indicates that none of the predictors improve model fit.
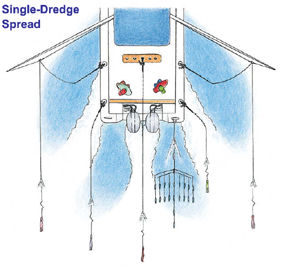
Get.models(selec_Coleop, subset = delta < 2)īarplot(t(imp_Coleop), main="Coleoptera") RegMODEL_Coleop <- lm(SR_Coleop_Mac ~ Area + Age + Altitude, SR_Coleop_Mac <- log10(species$Coleoptera + 1) Species <- read.table("DataSpecies.txt", h=T)
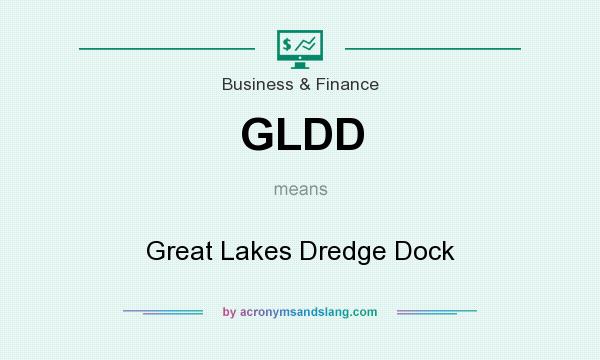
This is the input: biogeo <- read.table("DataIslands.txt", h=T) I need find which is the best model / variables for explaining the species richness of Coleoptera in Hawaii islands.
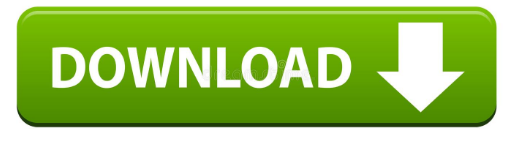